DeepSec 2023 Press Release: Language Models do no cognitive Work –
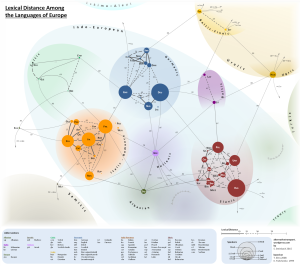
Lexical Distance Map (Graphic: Stephan Steinbach, alternativetransport.wordpress.com)
The term Artificial intelligence (AI) is in the media, but it consists only of language simulations.
If one follows the logic of the products currently offered under the AI label, we could easily remedy the shortage of skilled workers in the information technology sector. Take random people and let them consume tutorials, code examples, training videos and other documents related to the field of application for a few months. After this learning phase, skilled workers would automatically be available. TThe DeepSec conference is asking why there is still a lack of qualified personnel in IT. Algorithmically, the problem already seems to have been solved.
Large Language Models (LLMs) and AI
The so-called generative AI, which is now on everyone’s lips, is mathematically assigned to the research field of artificial intelligence. GPT, LLaMa, LaMDA or PaLM are models Google, Meta and OpenAI (supported by Microsoft) have thrown onto the market that. There are other models that are freely available or limited. All of them are so-called Large Language Models (LLMs). The enormous size of learning data used for training characterises this class of algorithms. Through questions, also called prompts, the algorithms then compose an answer from parts of the learned data. To do this, the models analyse the patterns of the languages they have “learned”. It then generated a shortened mixture of the learning data, which is output in well formulated language. This is then the answer to a question. It does not create new knowledge or insights, nor is there any analysis of the content.
Speaking and writing is not thinking
LLMs neither simulate nor perform a thinking process. The output sounds good and can also be syntactically correct (for example, when code is generated instead of speech). They are digital parrots with a very large vocabulary. There is a scientific publication on this entitled “On the Dangers of Stochastic Parrots: Can Language Models Be Too Big?”, which critically questions the size of the learning data used for the models. The question also looks at the risks of the technology and how to address them. The authors conclude that the race for ever more complex language models and collections of learning data has only limited benefits. Running the algorithms in the state after learning has financial and environmental costs. The results of the effort are unpredictable (all models published and used so far are prototypes, i.e. an experiment). The size and complexity makes it difficult for researchers to access for scientific analysis. In addition, the high eloquence of the answers of the LLM algorithms means a risk regarding thoughtless adoption of misinformation.
LLM algorithms have hallucinations
There are other studies that have looked at the output of false information from the language models. On the one hand, the cause is the age of the learning data. GPT-4 has only learned data up to September 2021. All information that is younger is not part of the corpus and cannot be used for answers. Other reasons are inconsistencies in the learning data, inaccuracies in data collection before the learning process or incomplete sources. All these factors can lead to generated answers that are at best misleading or at worst simply wrong. There are no simple solutions to the problem. This means that currently incorrect answers, when they become known, are subsequently filtered out by adjusting the configuration. This is done by the providers of the language models. The operation of the models thus becomes more and more complicated over time because new filters are constantly being added.
Reference to information security
Generative AI algorithms are also to be used in information security. One focus there is the filtering of information from large amounts of data (the learning data). Their use sounds tempting, but the results must not be part of a hallucination, because answers to security questions must make clear and reliable statements. At this year’s DeepSec conference, the advantages and disadvantages of LLM algorithms in the context of security will be discussed. One use case is to support the analysis of security incidents. In these scenarios, one has a lot of data to evaluate, where linking patterns in the data collections can be helpful.
Programmes and booking
The DeepSec 2023 conference days are on 16 and 17 November. The DeepSec trainings will take place on the two preceding days, 14 and 15 November. All trainings (with announced exceptions) and presentations are scheduled as face-to-face events. For registered participants, there will be a stream of the lectures on our internet platform.
The DeepINTEL Security Intelligence Conference will take place on 15 November. As this is a closed event, we ask for direct enquiries about the programme to our contact addresses. We provide strong end-to-end encryption for communication with us: https://deepsec.net/contact.html.
Tickets for the DeepSec conference and trainings can be ordered online at any time via the link https://deepsec.net/register.html. Discount codes from sponsors are available. If you are interested, please contact us at deepsec@deepsec.net. Please note that we depend on timely ticket orders because of planning security.