DeepSec 2024 Press Release: The limits of ‘AI’ language models lie in security. DeepSec warns: ‘AI’ language models generate content and override authorisations
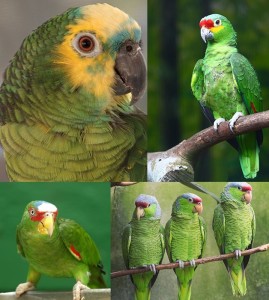
Amazon parrots are language generators (Image: Wikipedia)
Language model algorithms, also known as generative artificial intelligence, continue to celebrate their supposed triumphant advance through many media platforms. Security researchers have analysed the products and revealed a number of weaknesses in the ‘AI’ applications. This year’s DeepSec conference is dedicated to the threats posed by ‘AI’ learning models that use incomplete restrictions to analyse public and sensitive data.
Large Language Models (LLMs) as Auto-Completion
The technical description of the many ‘artificial intelligence’ (‘AI’) products on the market is impressive. In simple terms, the concept behind the advertising campaigns consists of algorithms that copy as much data as possible, break it down and then recombine it to provide answers to any questions. The learning process when creating the language model is not initially monitored or moderated. Only in later phases does so-called ‘fine-tuning’ come into play, which compares questions with correct answers by random sampling. Certain words and statements are given meaning through statistical effects because the language model composes the answers from patterns that sound plausible. Reformulations of the question influence the answer.
However, a random component always remains as an influencing factor in the process. In 2021, this fact was investigated by researchers. The result with an overview of these stochastic processes was published in the article entitled ‘On the Dangers of Stochastic Parrots: Can Language Models Be Too Big?’. The study raises questions about the benefits versus the costs. Training large language models consumes a lot of energy and memory. The error rate cannot be easily corrected because the data from the learning phase cannot be easily corrected like in a database. The question arises as to what the current systems are worth to potential customers if their error rate is in the double-digit percentage range (sometimes over 50 per cent error rate). Current LLMs can therefore not be used for critical decisions in particular. Nobody would agree to an operation that a surgical robot would only perform correctly with a 50 per cent probability.
Unrestricted Copying of Data as a Security Vulnerability
The learning data of the language models is a critical point. Some manufacturers of the ‘AI’ models subsequently admitted that any content was used for the learning phases without regard to copyright and usage rights. The older models still have traceable documentation. Neither the training sources nor the costs for the learning phase are published for models GPT-3.5 and GPT-4. The abbreviation GPT stands for the term ‘Generative Pre-trained Transformer’. This means that the algorithm ‘generates’ content based on the learning data (by mixing it with pre-generated content). The corpus of learnt data is transformed by the algorithmic processing into a form that makes editing impossible. This means that sensitive data can no longer be specifically deleted or edited after the learning phase. This aspect is a problem for information security, as all services that subsequently learn can carry sensitive data out of an organisation. The fact that the LLMs cannot be operated locally and must be connected to a cloud platform introduces gaps in authorisation control.
Even local learning data harbours the risk of sensitive content being captured in an uncontrolled manner and then being used to obtain information by asking the right questions. This is a threat posed by the new Copilot+ Recall product for the Windows platform, for example. The feature takes a screenshot every second, captures the data visible on the desktop and stores it in a local database in a searchable format after being processed by an algorithm. This means that future attackers can simply search for this data back and analyse the information it contains. The learning algorithm has already removed the authorisations. The feature opens the door to espionage and misuse.
Attacks on Language Models
The vulnerabilities of language models mentioned at the beginning pose a further threat. Security researchers have already repeatedly succeeded in overriding the protective mechanisms of ‘AI’ tools by reformulating them. This involves providing information on how to build a bomb, disclosing sensitive information or other blocked content. One researcher has succeeded in getting all language models on the market to generate meaningless answers using certain queries. A publication on this topic is forthcoming. In addition, all ‘AI’ language models suffer from so-called hallucinations. This effect in the algorithms used has been known for over 20 years.
In the case of language models in particular, this involves information that is completely unrelated to reality. They are created by transforming the ‘learnt’ content. In the case of ‘AI’ algorithms that generate images, these effects can be easily recognised as additional visible fingers, duplicated features or objects inserted without reference. Hallucinations are an inherent part of the models due to statistical effects. Reduction can only be achieved through supervised learning and human feedback. For cost reasons, filters are built in instead that do not allow certain answers (but this can be circumvented by reformulating the questions).
These vulnerabilities are particularly critical for language model responses that are used in decisions or programme code. It is therefore important not to use any of the current zoo of ‘AI’ tools unsupervised and to check the responses accordingly. The DeepSec conference will focus on such attacks and the resulting risks for applications. The call for papers is already open and runs until 31 July 2024.
Programme and Booking
The DeepSec 2024 conference days are on 21 and 22 November. The DeepSec training sessions will take place on the two preceding days, 19 and 20 November. All training sessions (with announced exceptions) and presentations are intended as face-to-face events, but can be held partially or completely virtually if necessary. For registered participants there will be a stream of the presentations on our internet platform.
The DeepINTEL Security Intelligence Conference will take place on 20 November. As this is a closed event, please send direct enquiries about the programme to our contact addresses. We provide strong end-to-end encryption for communication: https://deepsec.net/contact.html
Tickets for the DeepSec conference and training sessions can be ordered online at any time via the link https://deepsec.net/register.html. Discount codes from sponsors are available. If you are interested, please contact deepsec@deepsec.net. Please note that we are dependent on timely ticket orders due to planning security.